Real-time Driving Behavior Profiling Using UTDrive-MOBILE-App
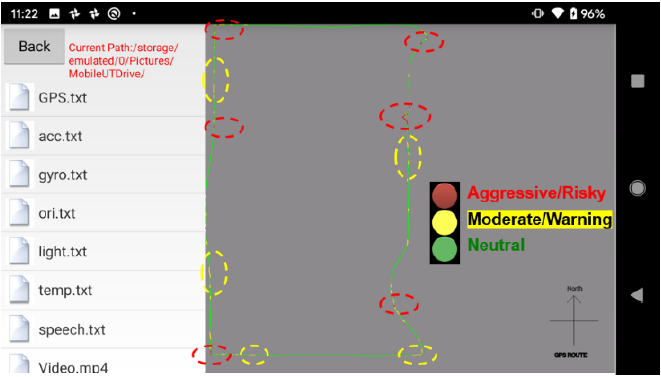
This project developed an advanced driving behavior model that combines driving behavior, traffic conditions, and driver psychology which are expected to improve the understanding of driver behavior from both micro and macro views. More details…
Image-based Techniques in Driving Performance Analysis
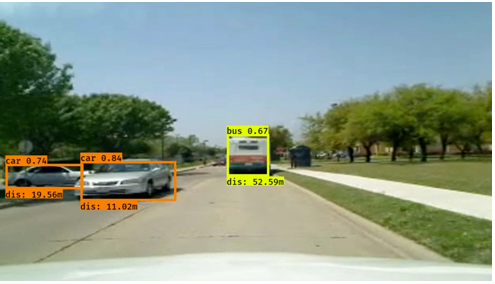
In this research, we studied driver performance with the help of image-based information. Driver assessment has been done based on driver’s following distance to other vehicles and pedestrians and off-center lane rates. More details…
Risky Lane Change Recognition
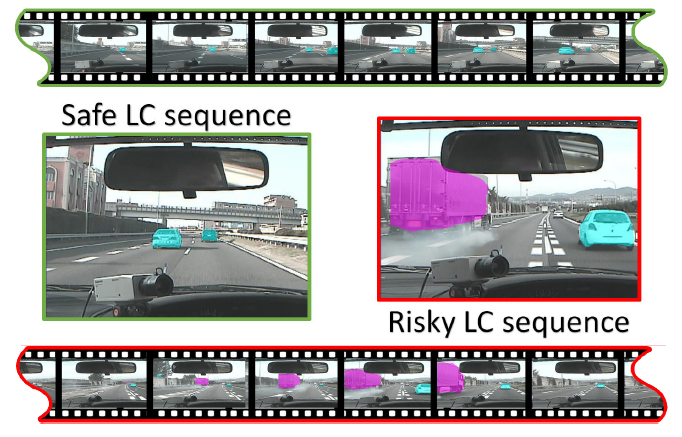
This project is collaborated with Dr. Yurtsever and Behavior Signal Processing Lab in Nagoya University. A novel deep spatiotemporal framework with segmentation mask transfer for risky action detection in short lane change video clips was proposed. This approach illustrated the adaptive capabilities of transfer learning in the intelligent vehicle domain. More details…
Driving Event Recognition using Free-Positioned Portable Device
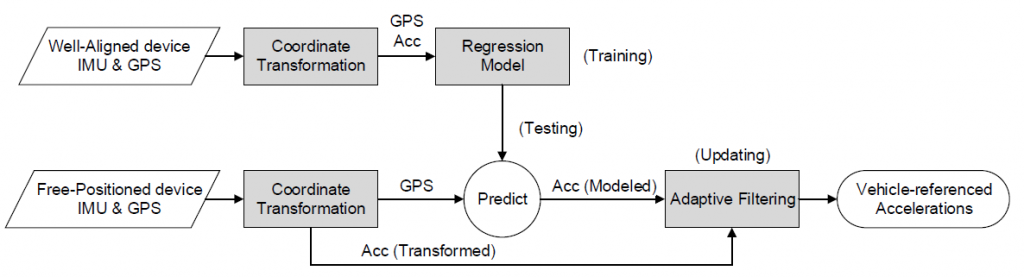
This project explored a solution of converting the smartphone-referenced IMU readings into vehicle-referenced accelerations, which allows for a free-positioned device for in-vehicle dynamics sensing and other applications. More details…
Driving Performance Analysis

It would be worthwhile to consider vehicle dynamic signals obtained from theController Area Network (CAN-Bus) and smartphones to analysis the effect of driver experience and vehicle familiarity for issues in driver modeling and distraction. More details…
Analysis on Driver Distraction
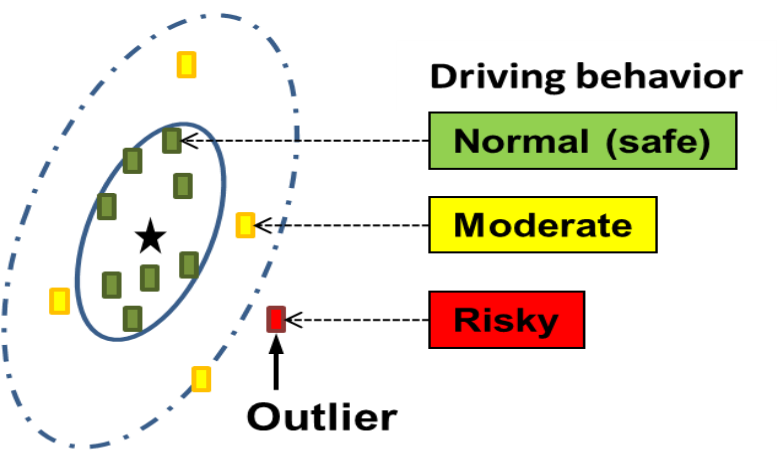
All driving patterns are clustered into several distinctive regions. The deviations from normal executions that are reflected in feature space are considered distracted or abnormal driving. This approach is applied to analyze the secondary task influence on driving. More details…
Driving Maneuver Recognition
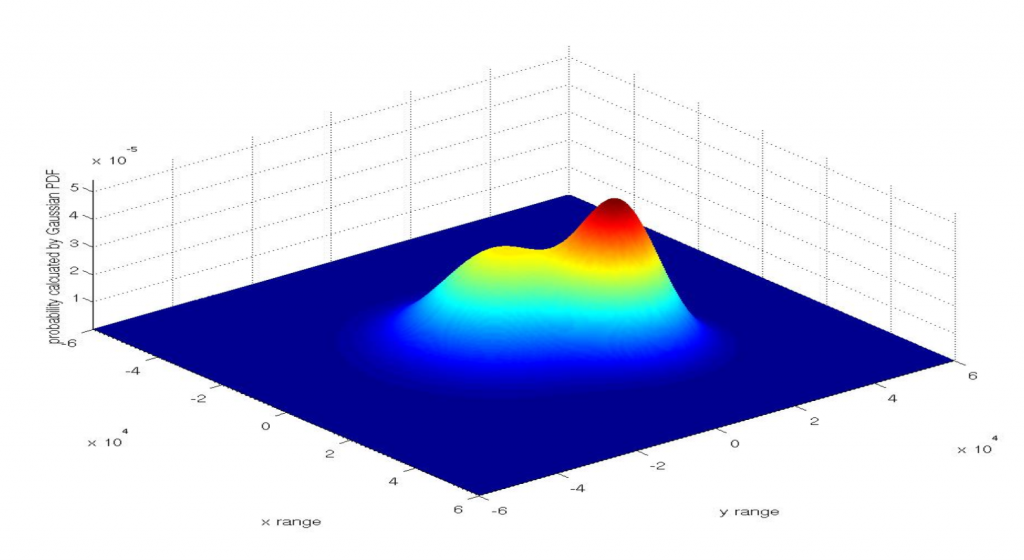
Stochastic models are used to recognize the maneuvers and routes in a predictive manner. Understanding how these maneuvers are performed can provide information on how the driver controls the vehicle and how driving performance varies over time. More details…
Portable Data-Collection Platform
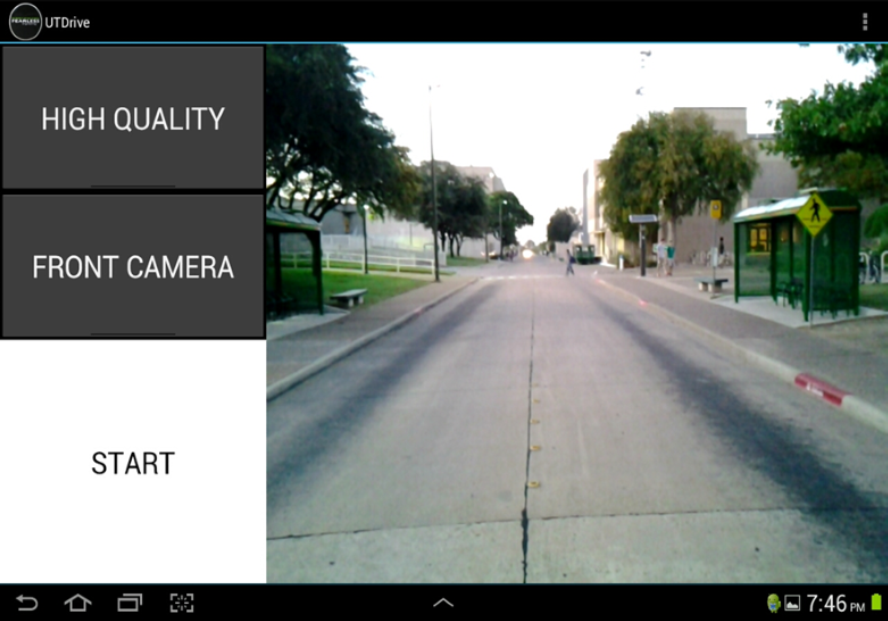
Mobile-UTDrive, is an Android based smartphone/tablet app, which is leveraged as a cost effective approach for in-vehicle data collection, monitoring, and added safety options/feedback strategies. More details…
Human-Centric Active Safety Systems
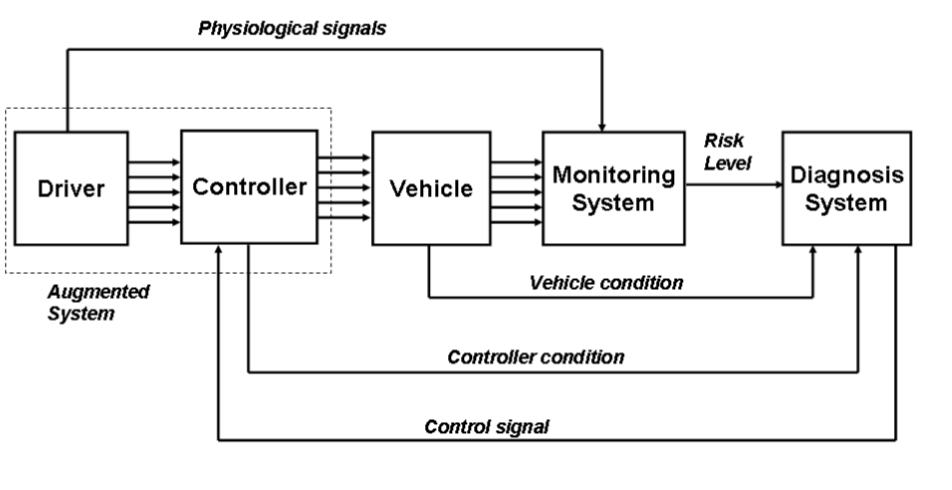
Driver behaviour models are used to design context-aware, human-centric active safety systems. More details…